About ADFM 2024
The rapid advancement of foundation models in fields like healthcare, cybersecurity, and finance highlights the urgent need to improve their anomaly detection capabilities. Despite their growing application in high-stakes areas, the challenges of using these models for anomaly detection remain underexplored. The Anomaly Detection with Foundation Models (ADFM 2024) workshop aims to address this gap by focusing on the intersection of foundation models and anomaly detection. Our organizing and technical committee, composed of leading experts, provides a platform for advancing research and discussing the recent breakthroughs, and the technical and ethical implications of deploying these models. ADFM 2024 will foster interdisciplinary collaboration and contribute to the development of more reliable and effective anomaly detection systems in artificial intelligence.
Where
International Convention Center Jeju (ICC Jeju), Jeju Island, South Korea
When
August 4, 2024
Keynote Speakers
[More info about keynote speakers will be updated here]
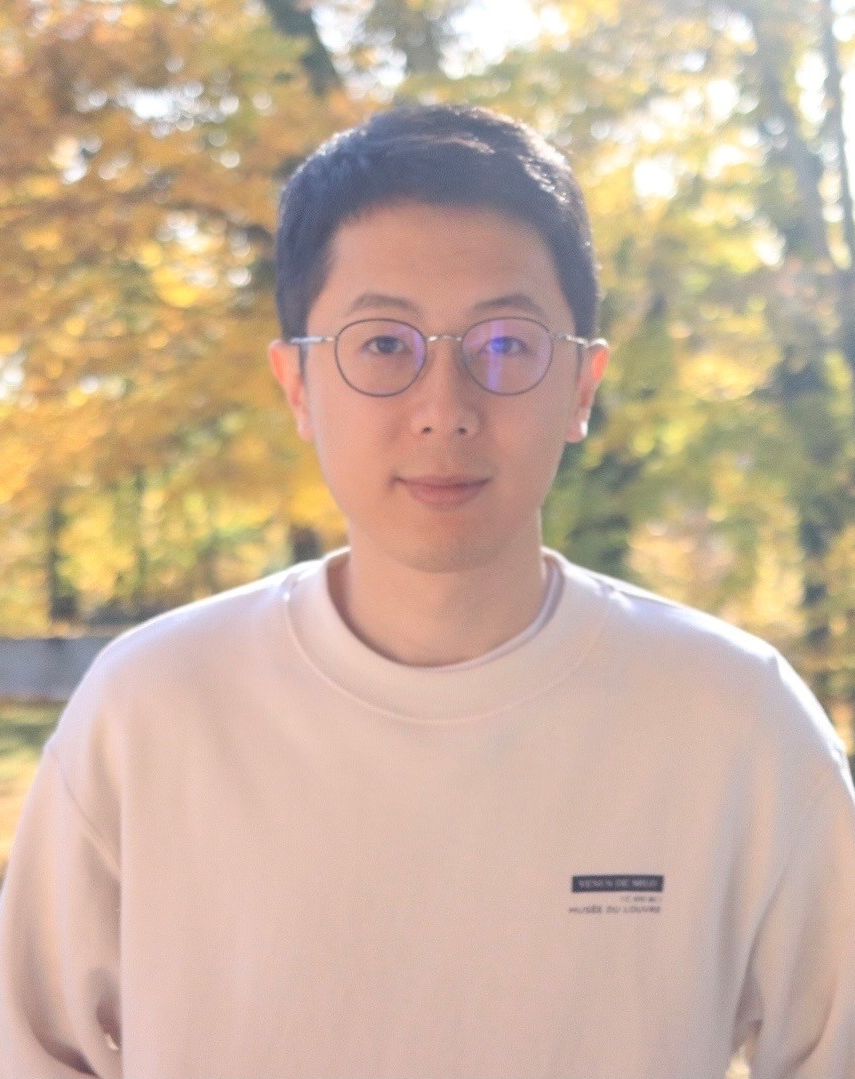
Chen Qiu
Bosch Research
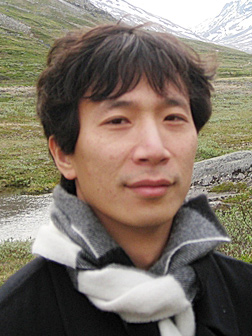
Jong-Seok Lee
Yonsei University
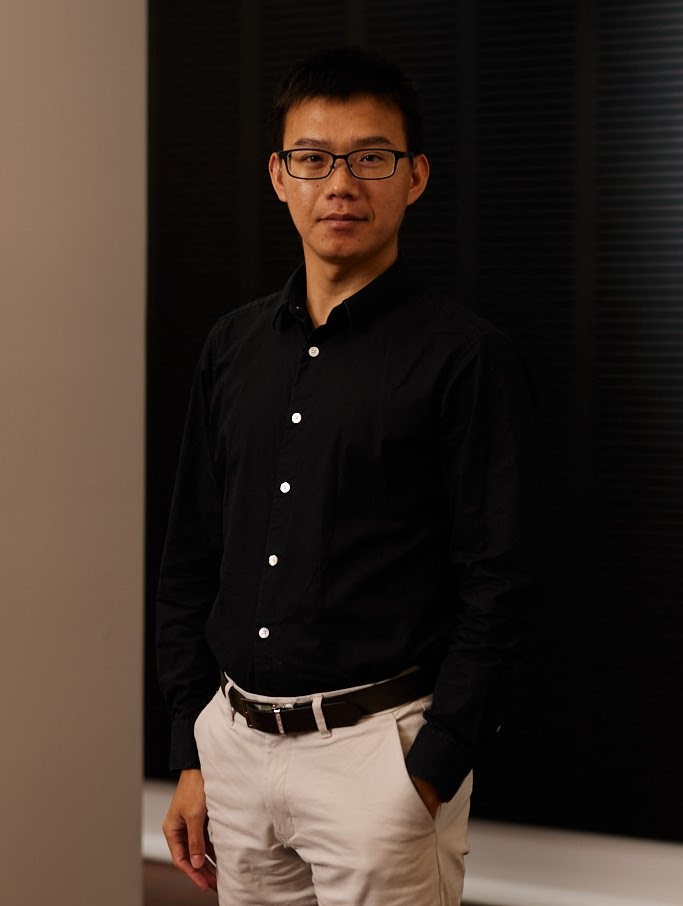
Guansong Pang
Singapore Management University
ADFM 2024 Schedule
[in Jeju local time]
[More info about the schedule will be updated here]
Submissions
Submission Instructions
We welcome full paper submissions. The papers must be no longer than 9 pages in total: 7 pages for the body of the paper and 2 pages for references. Please submit at the following CMT website:
ADFM 2024 CMT submission website.
The paper submissions must be in pdf format and use the IJCAI 2024 official templates. All submissions must be anonymous and conform to the IJCAI 2024 standards for double-blind review. The accepted papers will be posted on the workshop website and will not appear in the IJCAI 2024 proceedings. IJCAI 2024 workshop chairs enforce that at least one author of each accepted paper must travel to the IJCAI venue in person and present the paper, and that multiple submissions of the same paper to more IJCAI workshops are forbidden. Failure to comply with the aforesaid rules may cause the paper to be removed from the workshop program.
Submission deadline: April 26 May 10, 2024 AOE Time
Notification to authors: May 31, 2024
Camera ready deadline: June 7, 2024 AOE Time
We invite the submission of original and high-quality research papers in the topics related to anomaly detection with foundation models. Each accepted paper will be presented as either an oral, spotlight, or poster presentation.
Topics
The topics for ADFM 2024 include, but are not limited to:
- Foundations and principles of foundation models for anomaly detection.
- Innovative anomaly detection algorithms and frameworks leveraging foundation models.
- Application-specific anomaly detection using foundation models, including but not limited to finance, healthcare, cybersecurity, and industrial systems.
- Evaluation metrics and benchmarks for assessing anomaly detection in foundation models.
- Techniques for enhancing the explainability and interpretability of foundation models in anomaly detection tasks.
- Strategies for ensuring fairness and reducing bias in anomaly detection with foundation models.
- Privacy-preserving methods in anomaly detection using foundation models.
- Trustworthiness and reliability of foundation models in critical anomaly detection applications.
- Cross-disciplinary approaches for improving anomaly detection, incorporating insights from other fields such as psychology and sociology.
- Incremental learning and adaptation mechanisms for foundation models in dynamic environments.
- Integration of domain knowledge and expert systems with foundation models for improved anomaly detection.
- Exploring the limitations and challenges of current foundation models in detecting anomalies in complex and noisy data.
- Forward-looking perspectives on the evolution of anomaly detection methodologies with the advancement of foundation models.
ADFM 2024 Venue
Jeju
ADFM 2024 will be held at the International Convention Center Jeju (ICC Jeju), Jeju Island, South Korea in the afternoon on August 4, 2024.
Organizers
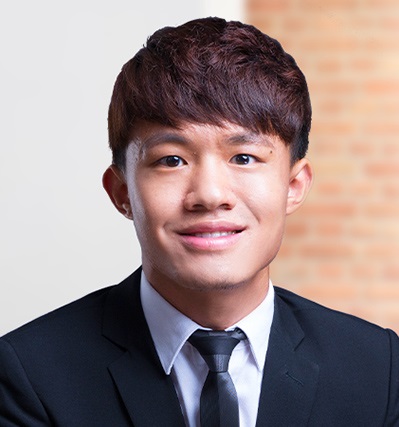
Ziyue Li
University of Cologne
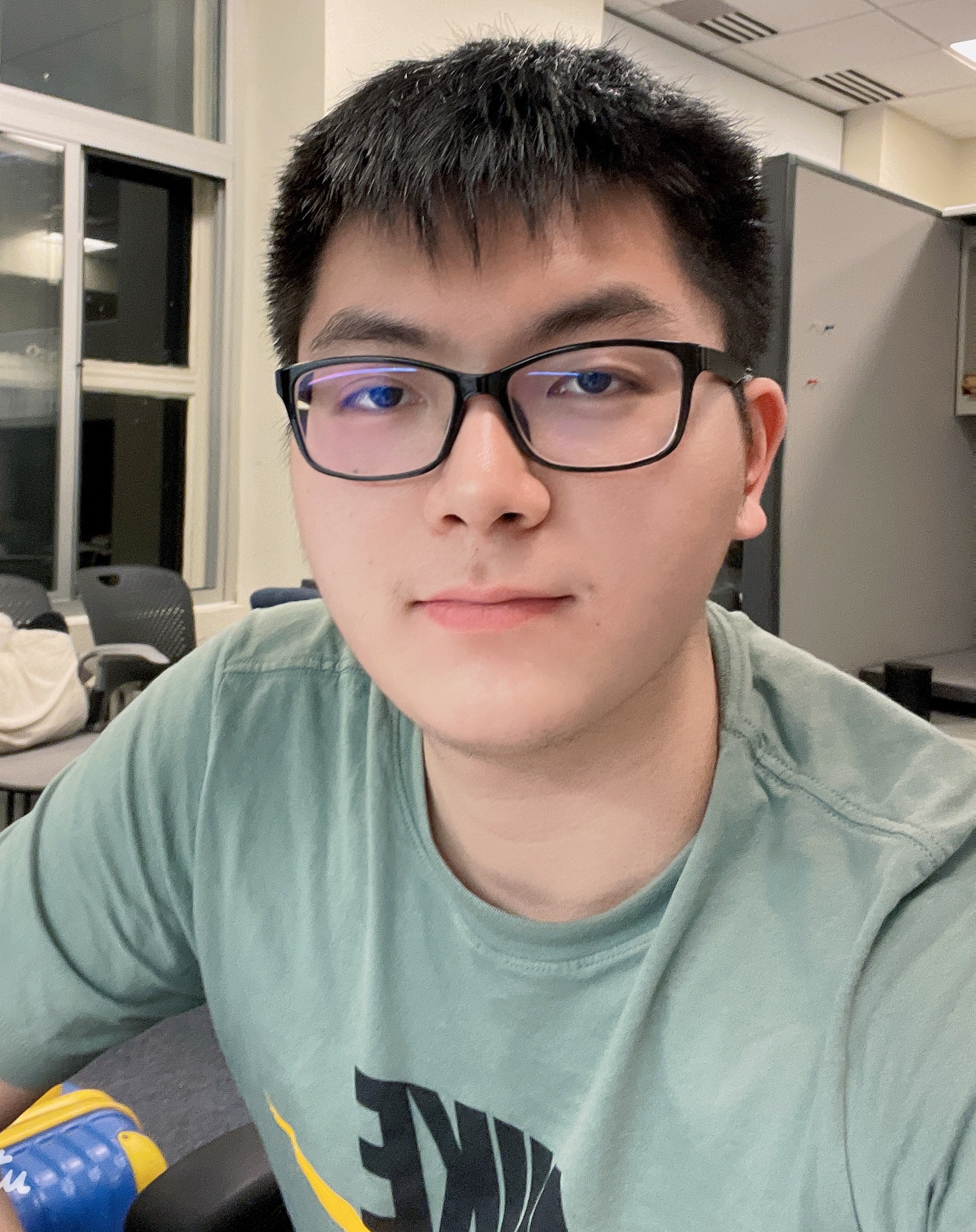
Yizhou Wang
Northeastern University
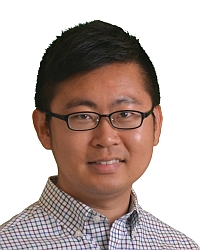
Kuan-Chuan Peng
Mitsubishi Electric Research Laboratories (MERL)